Assessment Control
The scientific method revolutionized discovery by introducing a reusable, adaptable framework for investigating natural phenomena. Guided by structured questions and systematic data collection, this approach enabled condition-based decision-making, and facilitated iteration, refinement, and testing of hypotheses. The one limitation? It only benefited the most disciplined and rigorous researcher. Could Specialized AI overcome the limitation and bring this power to every innovator and project team?
Yes, it does, and here is how. Model-based Cognition mirrors the scientific method through Assessment Control—an executable instance of reusable, adaptable, model-based knowledge within the system. Users engage through structured knowledge models, where each interaction conditionally can inform subsequent actions or queries. Iteration, refinement, and testing of concepts happens concurrently and in real-time. AurosIQ serves as a Copilot, assuming the responsibility for scientific rigor, freeing human assets to innovate and create.
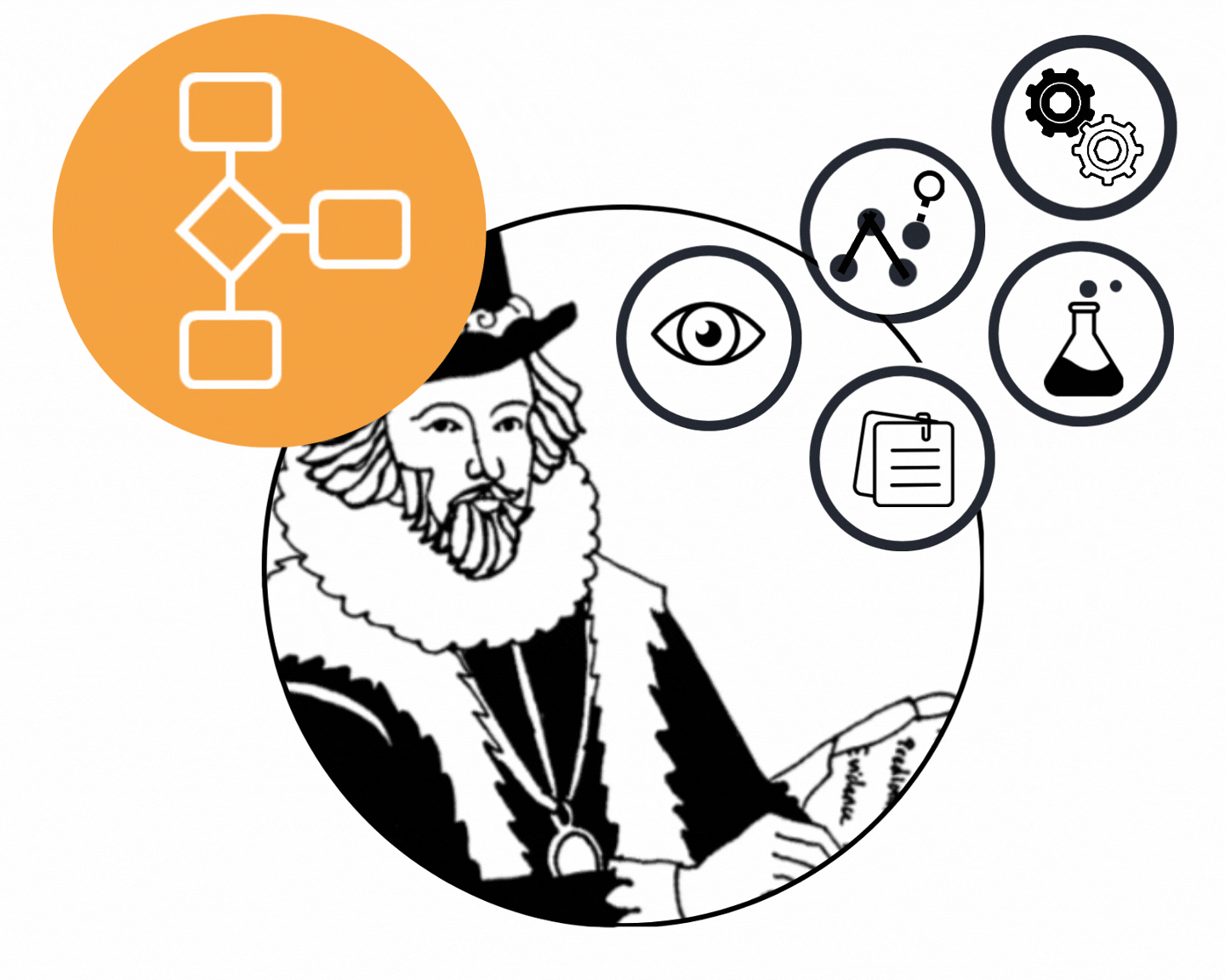
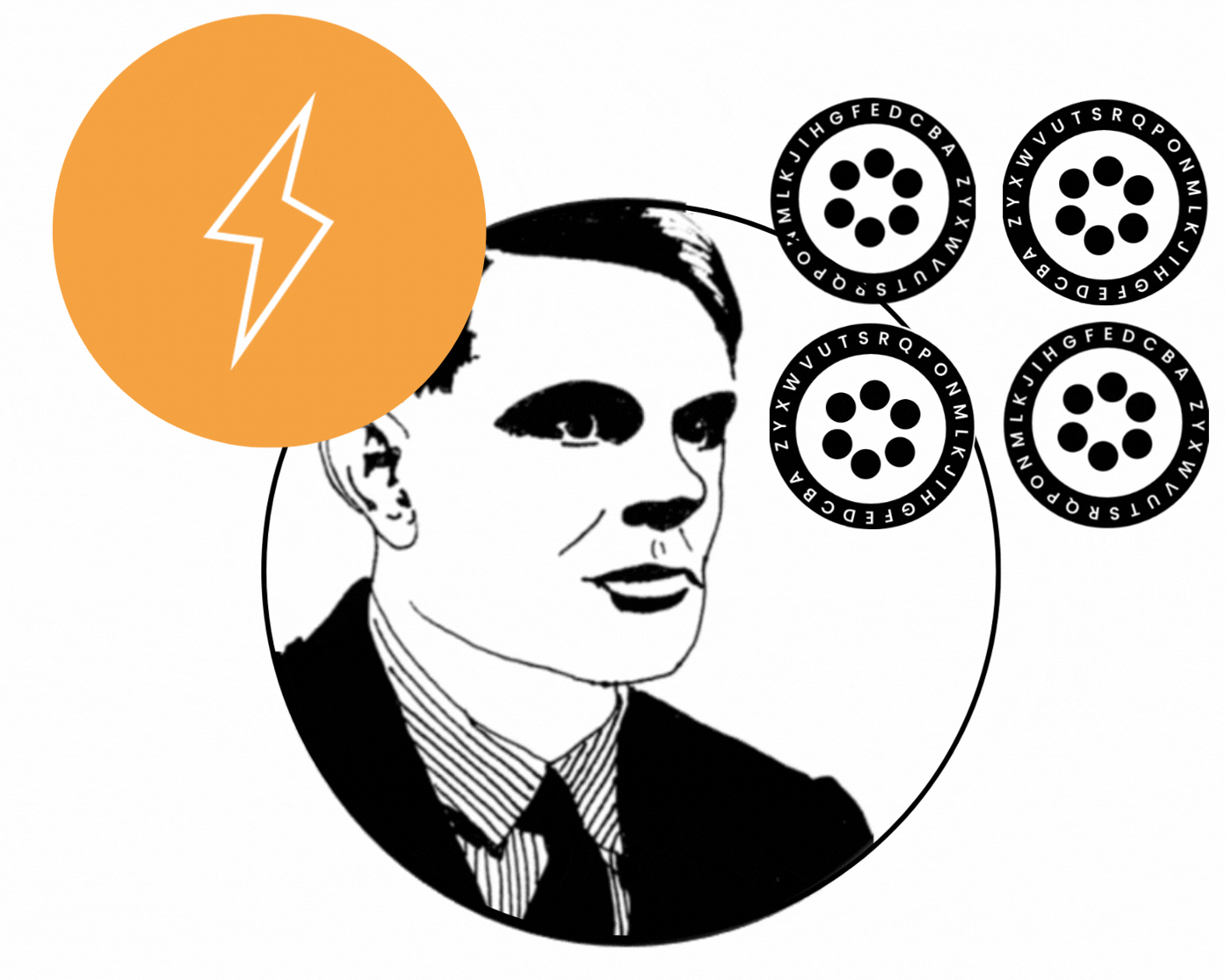
Knowledge Processor (Engine)
During World War II, a pivotal breakthrough in the effort to break the Enigma code was the development of a specialized machine known as the “Bombe.” This device leveraged known variables—partial, predictable elements from intercepted messages—and used circuit logic to deduce unknown variables, such as the daily rotor position and plugboard settings of the Enigma machine. With the Enigma’s astronomical number of possible configurations, manual decryption was virtually impossible. By systematically eliminating incorrect possibilities and identifying patterns, the Bombe transformed indecipherable communications into actionable intelligence, ultimately tipping the scales of war in favor of the Allies.
Model-based Cognition achieves similar success through its Knowledge Processor (Engine), which echoes the Bombe’s methodology. It can be applied simply – evaluating system status by testing executed variables against known requirements – or sophisticatedly by propagating these variables throughout the modeled system to deduce missing elements and automate conditional logic such as contingent steps, data or assessments. This automation eliminates human error, enabling AurosIQ to manage, at scale, the organically increasing complexity of the system, without compromising the quality or efficiency of execution.
Parameter Management
The Phoenician alphabet used a limited character set to represent sounds rather than unique symbols for every word/object. This simplified the creation, translation, and transcription of words leading to rapid and massive expansion of language and literacy. New and increasingly complex ideas and concepts could now be built by leveraging, combining, and improving prior knowledge. The age of specialization (which depends on learning and iteration) was born.
Likewise, Model-based Cognition uses Parameters as its “alphabet.” Parameters are the basic building blocks used to create discrete models which interact and interconnect. Increasingly complex models and systems of models emerge organically from the combination and recombination of these models with shared parameters. The management and evaluation of these parameters now becomes a strategic capability, which can be performed either deductively, during instantiation when an assessment is defined first, or inductively, where no pro-forma assessments exist and the system is being developed bottom-up.
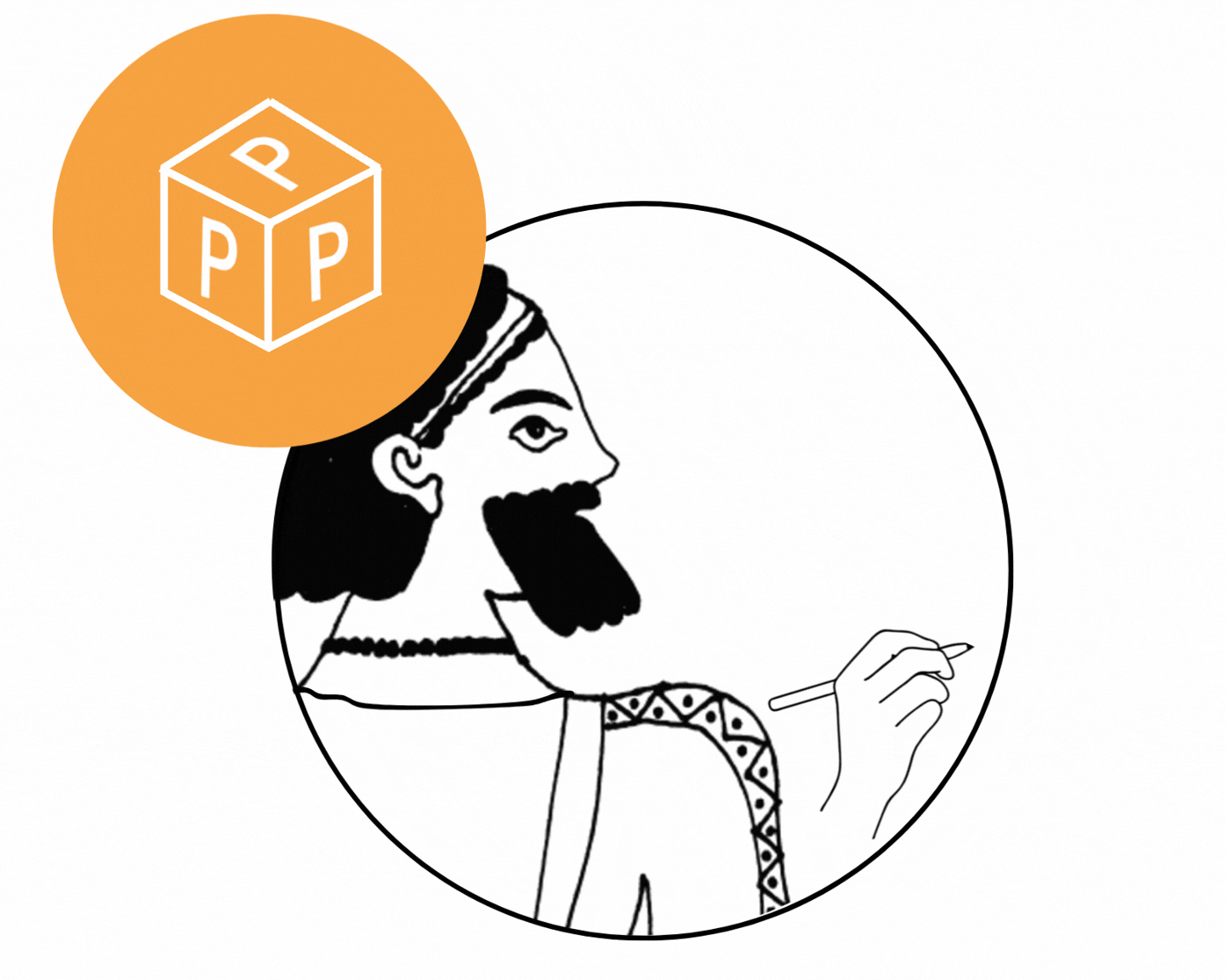
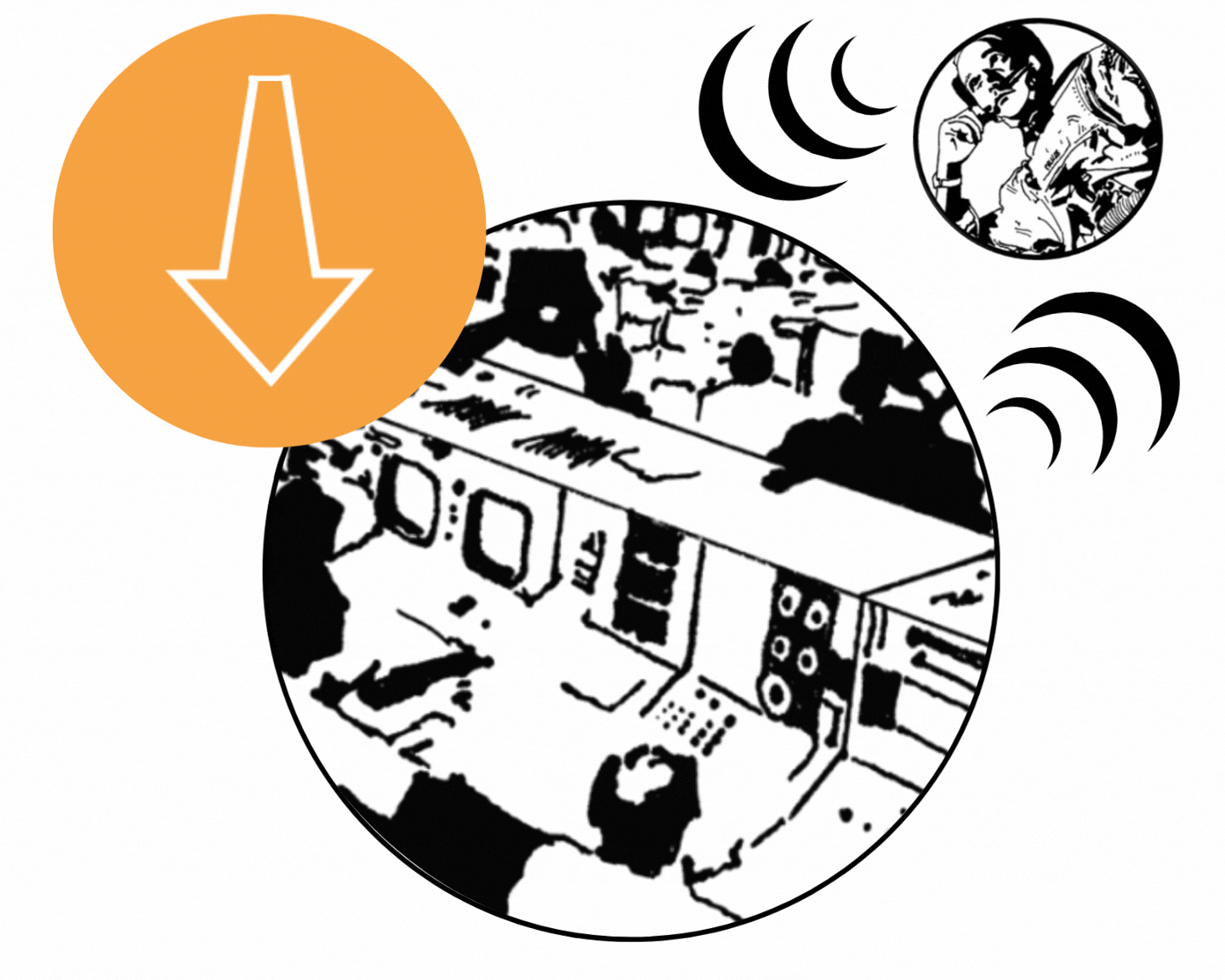
Dynamic Knowledge Provisioning
In 1969, NASA achieved the historic feat of landing on the Moon using computing power comparable to that of a graphing calculator— technology orders of magnitude less capable than today’s smartphones. NASA overcame seemingly insurmountable technical and operational challenges by using selective data transmission, which balanced limited capacity with operational priorities and spacecraft constraints. By transmitting only the most essential data NASA ensured the precision, safety, and ultimate success of the Apollo missions.
Similarly, Model-based Cognition achieves efficiency and success through Knowledge Provisioning; delivering precise context-relevant data if, when, and where it is needed. This approach liberates both mechanical systems and human operators to focus entirely on utilizing the information in the flow of work, rather than searching for or interpreting said information. Just as selective data transmission was critical to the Apollo missions, Knowledge Provisioning is indispensable for ensuring optimal performance in today’s complex systems.
Service-Oriented Architecture
The Roman army achieved unparalleled dominance over the ancient world, consistently outmaneuvering and outlasting its adversaries. At the core of this success was its ability to seamlessly connect and integrate specialized units—such as archers, cavalry, engineers, logisticians, and infantry—into an integrated force. By rapidly adapting to shifting conditions and deploying these optimized units with agility and precision, the Roman legions secured their many victories through mastery of coordination and responsiveness.
Likewise, Model-based Cognition relies on a robust service-oriented architecture to connect and integrate with external data systems across an organization. This architecture ensures that information—such as real-time data from a plant floor or input from advanced tools like VR headsets—is seamlessly conveyed into the Model-based Cognition framework. By integrating this information, the system delivers actionable insights directly to mechanics, designers, or operators, enabling fast, informed decision-making. Just as the Roman army’s adaptability relied on efficient integration, Model-based Cognition transforms connectivity between specialized units into a core strength, ensuring that every layer of an organization is equipped to respond dynamically and effectively.
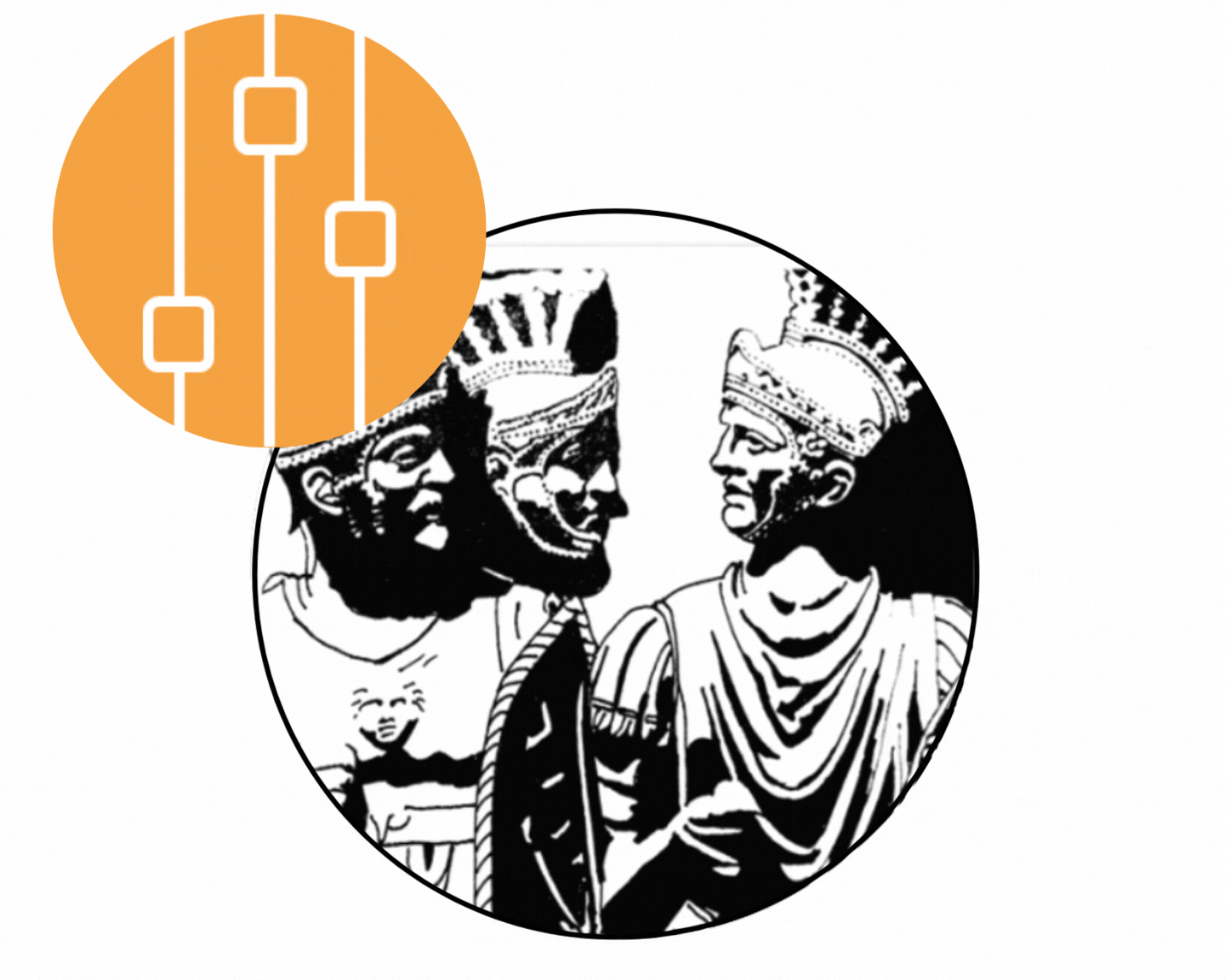
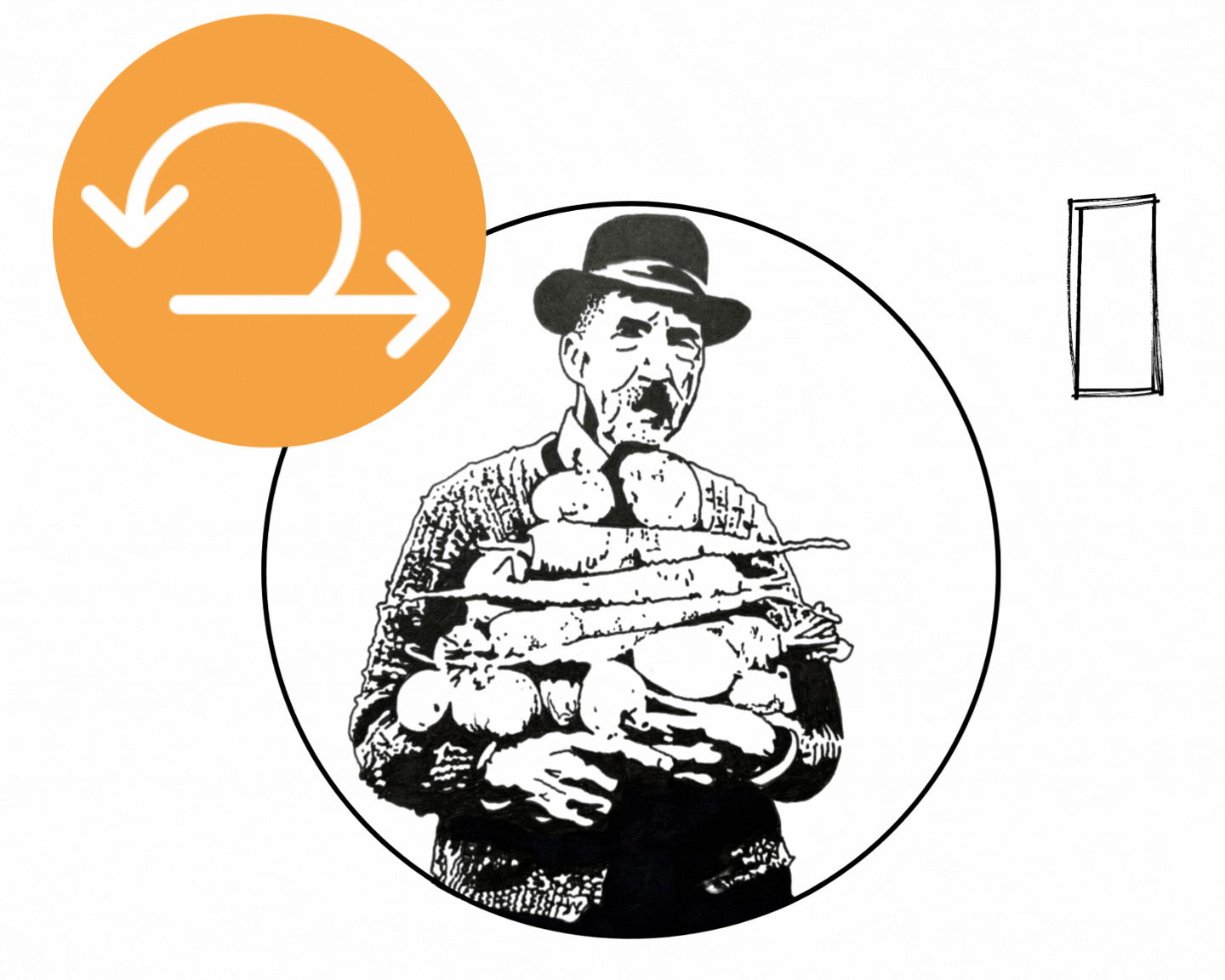
Learning Cycles
Complicated systems (like a car or a Swiss watch) are defined by deterministic variables and can be diagnosed and repaired with predictable outcomes. Alternatively complex systems are affected by stochastic variables with nonlinear interactions and can’t be predicted or controlled—only guided and influenced. Consider a garden – Seasonal and annual variations in climate, pestilence, and soil all add stochastic variability. Gardens thrive not through rigid control but through attentive observation, pattern recognition, and thoughtful application of new insights. Gardeners learn from both their successes and failures, gradually refining their methods. Each planting season builds upon the knowledge gained from the last, fostering an ongoing cycle of growth and improvement.
In a similar way, Model-based Cognition employs iterative learning cycles to drive progress. By identifying patterns and uncovering areas for improvement, it generates actionable insights for review and reuse. This continuous process of self-assessment and adaptation ensures the system remains dynamic and resilient, enabling sustainable and measurable growth over time.
.
Let’s Connect!
Learn how Auros IQ can change the way you work. Maybe you’re curious about how our software can solve your unique challenges, or you’d like to learn about its ease of adoption — we can help!
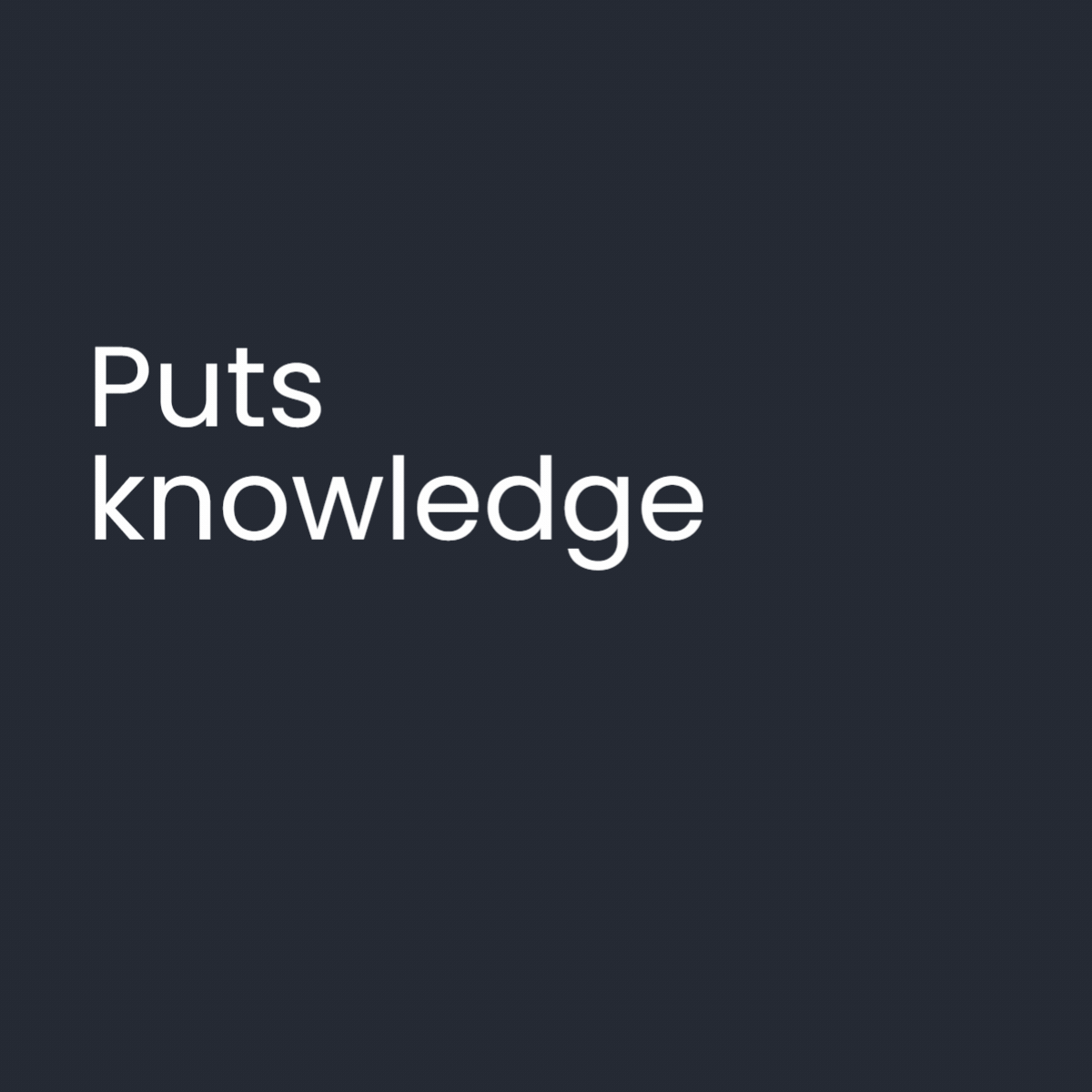